by Dot Cannon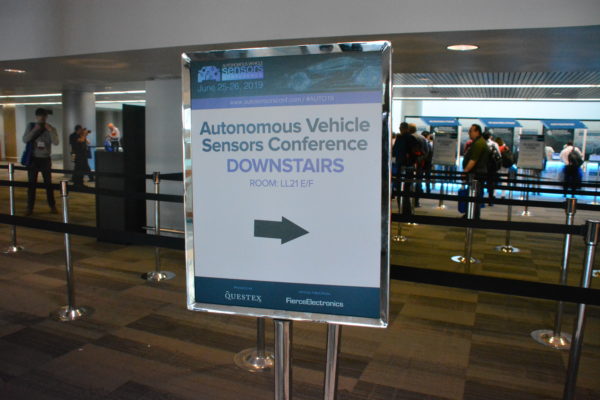
“I think it’s a very exciting time to be involved with modern automotive,” said Autonomous Vehicle Sensors Conference Chair Willard Tu in his opening remarks on Tuesday morning.
As the second annual Autonomous Vehicle Sensors Conference began, at San Jose’s McEnery Convention Center, Will, who is Senior Director of XILINX Automotive Business Unit, outlined what the audience could expect.
“We’re going to have Uber come up and give a keynote and kind of frame the day for us,” he said.
“We’re going to talk about AI–what’s really happening with AI.
And he asked the audience to start thinking.
“What is the best sensor that you need? I’d really love you guys to come up with some questions for our panel of sensor experts.
He also pointed out a different area which the day’s speakers would cover.
“A lot of the time, (sessions are about ) exterior sensing. This afternoon is going to be more about interior sensing. In-cabin sensing is very, very important.”
The Autonomous Vehicle Sensors Conference, which runs concurrently with Sensors Expo and Conference‘s pre-conference symposia, has grown from a special session in 2017 to a one-day 2018 event. This year, it spans a day and a half.
And Tuesday’s keynote speaker, Dr. Amrit Sinha of Uber Global Supply Chain and Business Operations, started his presentation, “Uber’s Path to Autonomous Vehicles”, with an intriguing parallel.
History and Uber
Dr. Sinha told the attendees of “The Great Horse Manure Crisis of 1894.” In the 1890s, he said, the proliferation of horse-drawn vehicles led New York and London to worry that their city streets would be buried under horse droppings.
“Here’s a photo of Easter in New York City in 1900,” he said, displaying a slide with one lone automobile surrounded by horse-drawn carriages.
“(But) just in the next 15 years, this was reversed. You’d be hard-pressed to find a horse…in New York City.
“Today less than one percent of people in the U.S. own horses. I see a very similar future for personally-owned cars in the U.S.”
“Uber services more than 75 million people annually,” he continued. “It took us five years to get our first million rides. In the past year, we got a million.”
Dr. Sinha outlined the services Uber currently offers–including their Jump bicycles and Moto motorbikes, for cities like Bangkok . He also discussed their vision for the future. Among future services will be Uber Elevate.
“Elevate is for cities growing vertically,” he said. “(They’ll be using vertical takeoff planes for cities like Dallas and Los Angeles).”
Currently, he continued, Uber moves “people, freight and food”. Due to the fragmentation of the freight industry in the U.S., Uber is a viable option for businesses. Meanwhile, food and transportation are mainstays.
“Uber Eats works with more than 160,000 restaurant partners. (And) Eats doubledips into the rideshare business because the delivery partners are Uber drivers.”
Reaching for autonomous
But despite these business models, Dr. Sinha said, there was a definite gap.
“Our penetration is just one to two percent. We have to do a better job of scaling. That’s exactly where autonomous comes in.
“Uber is not in the business of making cars,” Dr. Sinha added. “That’s exactly why we need a team (creating self-driving vehicles).”
Dr. Sinha addressed the issues facing different types of sensors on an autonomous vehicle.
Displaying a slide of a custom SE-90 car, with 360-degree radar coverage, he explained, “Cameras (are) the best way for how we see the world (but darkness is a problem. Radar can’t detect potholes; smoke, fog or dust affects visibility with LIDAR.)”
“There’s always room for more sensors, like thermal…at Uber, we believe we need to solve autonomy first before ruling out (sensors that may seem extraneous).”
“…The road to self-driving is neither easy nor short. I think we have (the ability to get there).”
“So my humble request to the sensor community, is to keep pushing the envelope and give us the most awesome sensors you have (on a foundation of safety). I think we have the power to make that change happen, just like the Great Horse Manure Crisis didn’t happen.”
Exploring the barriers
Next came a look at some of the challenges currently facing the construction of autonomous vehicles.
Co-hosted by Willard Tu and XILINX DIrector of Product Marketing Nick Ni, the presentation was entitled, “AI Challenges on AD Development: Evolution or Technology Revolution?”
“Central compute, processing, a lot of learning (is) going on,” Will said. “There’s not one way to do it. Everybody’s learning rapidly as we go through this process.”
“The question is, how do we take it into a real commercialization deployment,” Nick said. He named a number of future avenues, including drones.
“(But) if the market’s so huge, why don’t we see a huge amount of deployment? Because it’s very challenging.
Nick indicated that “one size fits all” would not apply to creating autonomous vehicles.
“How many of you have heard of DSA, or domain-specific architecture? ” he continued. “Nowadays, the workflow, such as AI, you need a specific architecture change for each (part) of the workload.”
Nick said every network had three areas that had to be customized: a custom data path, custom precision and memory hierarchy.
“The question is, what is the right precision,” he continued. “I believe the answer is, it depends.”
“If you create architecture that’s fixed, you’re always going to be giving up something. Because you’re not going to be running just one network.”
Artificial intelligence, he said, was evolving rapidly. But nothing, especially in the automotive field, was AI alone.
“Today, with Ai, it’s kind of pushing,” Nick continued. “We have to create separate chips to (perform different functions).”
Summarizing his talk, Nick told the audience that the automotive industry had not converged on a common approach to developing the AI for autonomous vehicles. Flexibility in the data pipeline was needed, he said, and so was adaptive silicon.
“The bottom line, it doesn’t matter how powerful your engine is. If you can’t pump the data fast enough, you’re wasting that resource.”
An analyst’s perspective
“There are two ways of autonomous driving,” said Yole Developpement Technology and Market Analyst Dimitrios Damianos.
“One is a car everyone can own. ..The second, a (robotic) car that cannot drive everywhere. So, the robotic vehicle is a disruption case.”
In his presentation, “Market Analyst View: Get the Relevant Quantitative Data on Autonomous Vehicle Sensors and Sensor Fusion”, Dimitrios displayed a slide of the five levels of autonomy.
(According to the NHTSA, these are: Level 1, some driving assist features included in the design; Level 2, partial automation; Level 3, conditional automation with the driver “ready to take control of the vehicle at all times with notice”; Level 4, high automation, with the vehicle capable of performing all driving functions under certain conditions but the driver may take control of the vehicle; and Level 5, fully automation, where the vehicle can perform all functions under all conditions but the driver has the option of taking control.)
A proportionate increase
Cost, Dimitrios said, was a major issue.
“Level One, six sensors cost $130,” he explained.
And the number of sensors keeps increasing by the level of autonomy. So does the price of the car.
“For robotic vehicles, you need a higher grade sensor,” Dimitrios continued. “With increasing level of automation, we will have more sensors inside the car…Each level has its own sensors, its own processing.”
Dimitrios explained that Level 1 has only some radar. Level 2 starts incorporating cameras, as well.
“When we jump to Level 3, we have LIDARs as well,” he said. “And then, Level 4 and 5 autonomy, fusion platforms are essential to use.”
…”Now, in 2019, we’re seeing some Level 3. We’re not talking about worldwide adoption because it will take some time before these levels will be affordable for the average consumer.”
But autonomous was still in the future, he added.
“The stakes are big. It’s for sure that bot of these markets will increase, because there is a need for autonomous driving to solve problems like traffic and pollution.”
Sensors and safety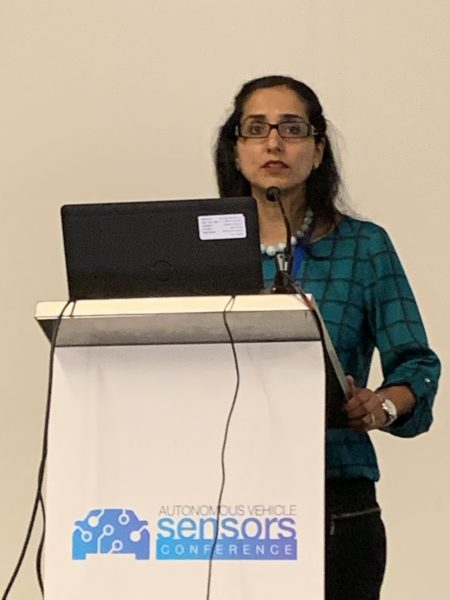
“By the time we get done with this, in the next one hour, we will have saved nine lives,” said ON Semiconductor Autonomous Driving Lead Radhika Arora.
She referenced a road traffic injuries figure from Boston Study Group, which showed ADAS preventing 28% of crashes. ON Sensors, she told the audience, were saving over 81,000 lives per year.
And in her presentation, “Saved by the Sensor: Vehicle Awareness and Exterior Sensing Solutions”, she would illustrate several ways her company was increasing the safety factor.
Degradation was one element ON Sensors was taking into consideration, Radhika said.
“Color filter erase degrades over time. So if you have a sensor in the windshield of a car there’s (sunlight) hitting that filter every day over weeks, months, years.
“What are we doing, from a sensors side, to address that? Our company works closely with pigment manufacturers…We adjusted pigmentation, ran tests.”
Functional safety was another area at which she and her team were looking closely.
Aspects of preparation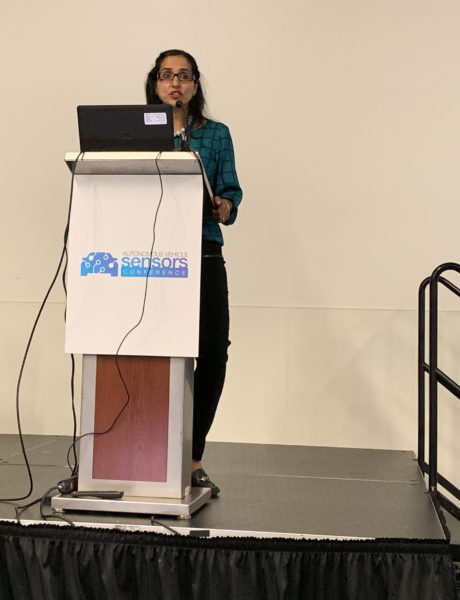
“When you look, do you see a fault in this image?” Radhika asked, displaying a slide of people and vehicles on a city street. (“Most people don’t.) There are pixel variances in the image which can be easily missed.”
“There are thousands of faults that we inject, we see how the sensors respond, and insert (technology) to serve as a backup in the event that feature fails.”
And cybersecurity was a third consideration.
“(A cyber) attack can happen in multiple ways. In the optical path, data path or the control path. And it’s important to be covered in all those data points,” she said.
“We understand the importance of high dynamics range. How would a sensor respond in conditions like that? We can plan for 99 percent of (the unexpected). That extra 20 db means everything.”
For increased safety, she said, autonomous vehicle cameras were trending towards more pixels and a wider field of view. Additional and more advanced cameras are also becoming more common.
“The motivation for more cameras is to get 360-degree view coverage,” Radhika explained. “Why more pixels? Road conditions, tire pressure, the road could be wet.”
Higher resolution radar, she continued, was necessary for Levels 3 and 4.
“You want to be able to not just detect the object, but to classify the object as well. When you’re talking about Level 5, you’re talking about cognitive radar, which is adding more ‘smarts'”.
Eyes for AI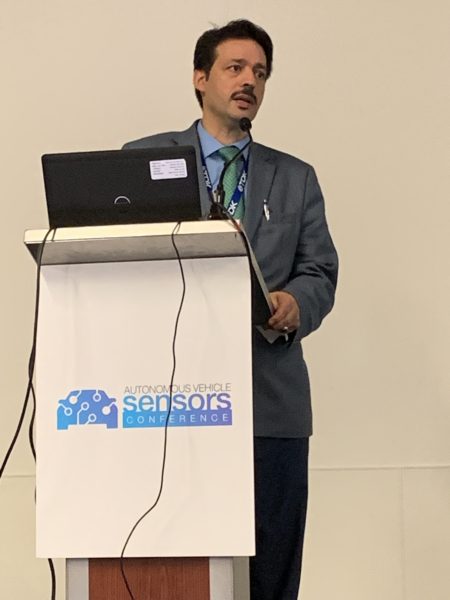
“Every sensor has its own positives and its own negatives,” said CalmCar CTO Dr. Faroog Ibrahim.
His presentation “AV Eyes: Front Camera Sensing For the Win”, illustrated how his company’s platforms maximize those positives to detect potential driving hazards.
“When we talk about training for AI, the accuracy from the camera is the best,” he said. “There’s stuff you can’t do with radar. Traffic signs, traffic lights, speed limit. The camera is the best solution for that.
“We do everything. When it comes to AI, you can do a lot of things.
“We are specialized in using single-camera technology.”
Dr. Ibrahim told the audience that CalmCar’s camera system can detect a pedestrian at 60 meters and track up to 80 meters, with a 92 percent accuracy rate. Traffic sign detection, lane detection and cyclist detection are all part of its front-camera system. In addition, it can detect vehicles approaching laterally.
“We are not saying that the camera system can do everything…but for every system, you really need to have fast detection,” he said.
“This (system) can make you understand the scene much better and you can get much better range in accuracy.”
Eyes on the driver
Another of CalmCar’s systems, he said, was the driver monitoring system. This particular system is designed to warn a driver who’s falling asleep or driving distracted. Its features include an eye closure warning, yawn warning and abnormal posture warning.
Dr. Ibrahim concluded his presentation was a video which showed how CalmCar’s systems work. Dr. Ibrahim showed the audience how his company one-camera system identifies vehicles on the road, detect traffic lights and free space. For example, it drew a large blue box around a bus, while smaller vehicles had differently-colored, smaller boxes.
“That’s all one camera. You can see how fast the detection happens,” Dr. Ibrahim said.
In the same video, the audience also had a chance to see how CalmCar’s driver monitoring system worked. In addition to drowsiness, the system monitored “Distraction 1 – including phone calls”, and “Distraction 2 – including smoking.”
The morning had flown by! And still ahead, after lunch, was a look inside the autonomous vehicles of the future.