by Dot Cannon
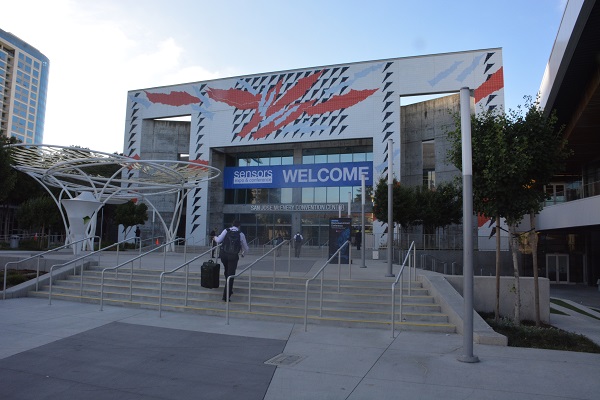
Attendees enter McEnery Conference Center for Sensors Expo 2018, San Jose.
New York is working towards statewide “smart” streetlights.
Farmers can now have sensor-equipped machines to separate the wheat from the chaff.
And human nature is something engineers have to take into account, when designing new products.
All of the above are insights from Sensors Innovation Week, a two-day free virtual event which Sensors Expo and Conference co-hosted with FierceElectronics on Thursday and Friday, July 16th and 17th.
Sensors Expo and Conference is the largest annual event dedicated to sensors, connectivity and the Internet of Things. 2020 marks the 35th anniversary of the show. However, due to the pandemic, the conference, which normally takes place in San Jose in June, has been postponed until November.
But the sharing of knowledge, current trends and new innovations continues. And on Thursday and Friday, Sensors Innovation Week spotlighted a number of new developments in the field.
Over the two days, the virtual event featured keynotes, fireside chats and discussion panels, in four separate sessions.
As noted in our previous post, the first sessions of Day One focused on the ways sensing technology is combating the pandemic.
The mechanics of innovation
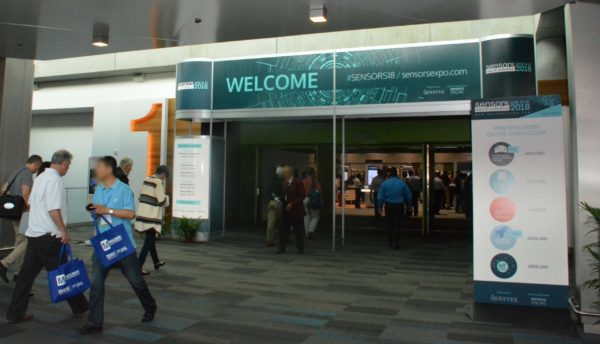
Entering the expo hall during Sensors Expo, 2018, San Jose.
During Day One’s second set of sessions, themed “You’ve Got the Power, Use it Wisely to Create Energy-Efficient Sensor Designs“, panelist and PowerRox Principal Brian Zahnstecher raised an interesting point.
“A lot of stuff boils down to basic human psychology, which is greed,” he said. “People always want more function, more application…battery life is an afterthought.”
The panel, which FierceElectronics Group Content Director Karen Field moderated, was exploring the challenges of sensor-related engineering design versus power efficiency. Brian’s comment summarized the core problem: we all want more, without considering how that happens.
The same issue had come up during a keynote entitled “How to Manage Power Wisely: Keep It Simple”, just prior to the discussion panel.
Interviewing Jetperch CEO Matt Liberty for the keynote, Karen asked, “Why is power (in sensor design) such an enduring challenge?”
“The problem we have,” Matt responded, “(is that power consumption is an aspect that touches on just about everything.). And as consumers we want more and more from our products.”
Using the example of a mobile phone, he continued, “What I can do with that phone is vastly different from twenty years ago.”
Power and life
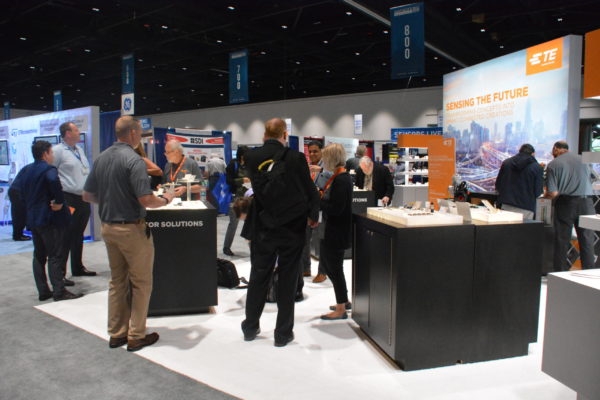
On the Sensors Expo floor, San Jose, 2019.
Karen brought up a “problem” that could actually be considered desirable: creativity. “Give engineers a little bit more power (when designing a device) and they’re going to figure out more features to add, ” she observed.
“A lot of times, reality gets in the way, “Matt replied. “You have a real deadline, a real project…so you create a real prototype…and it’s (difficult to retrofit for power efficiency).”
“I find it interesting that you talk about power as a feature,” Karen commented. “I think for many consumers (it’s a given).”
But efficiency in sensor-technology design, Matt said, required seeing power consumption as one of the device’s features. “(You need to ) ingrain it into your design and really, everyone (on the design team) has to be responsible for it. You really have to have a team that understands, what does (a device capability) do to power consumption.”
Matt predicted that, in the future, a lot of products on the market would be more focused on power efficiency. “I think we’re seeing much broader interest in low power design and broader interest in producing products (with) longer battery life.”
Troubleshooting the future
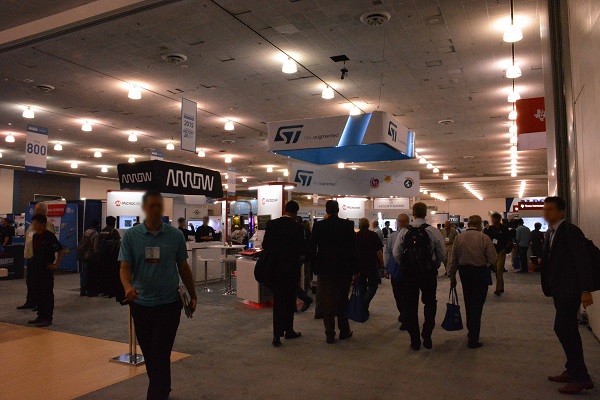
Attendees on Sensors Expo convention floor, 2016.
And the panel that followed, explored several power-versus-design problems, as cities become “smarter”.
“It’s not just a power limitation, but an economic limitation,” Brian said. “(Then, there’s the 5G electronic gap.). They’re installing new radios (on buildings) here in San Jose, and they’re blowing up transformers in the street. (With connected IOT 5G networks), we’re setting ourselves up for a very similar thing here, in this space.”
“I think good engineering starts with the use case, and (the value to the customer),” said Millibatt COO Leland Smith. “Instead of having hundreds of sensors broadcasting to a single receiver, you can have that power hop from sensor to sensor.
“I think with building sensing, there might be an opportunity to reimagine that a little bit.”
“I think when you look at building, one thing we should be looking at…(is) microgrids,” said CommScope Lead Market Development specialist Ronna Davis. “You can do energy harvesting (this way, recapturing the heat and feeding it back in).
“Microgrid (use) is growing at ten percent annually….Ten percent’s pretty big.
“…We can get there.”
City “smart” lights
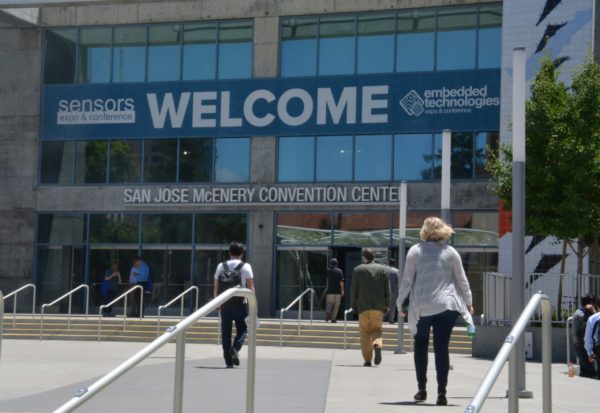
Attendees arrive for Sensors Expo and Conference, 2019, San Jose.
Day Two’s first series of sessions was themed, “Hey Network, It’s Me, Sensor!” These four presentations started with a keynote themed, “A New York IOT Success Story”. FierceElectronics editor Matt Hamblin served as moderator, interviewing New York Power Authority Key Accounts Manager Jesse Scott.
Matt and Jesse discussed NYPA’s current project: the implementation of more than one million “smart” LED streetlights, statewide.
Showing a short video, Jesse told the audience that NYPA will convert at least 500,000 streetlights to LEDs by 2025.
“Our vision is to make NYPA the first end-to-end utility in the country,” Jesse said.
Jesse explained that this goal isn’t just about LED lights. The streetlights will include sensors for additional functions. Weather sensing, public safety and license-plate reading are among those tasks.
“What’s your favorite IOT app?” Matt asked.
“Our basis for designing is that every light is connected, ” Jesse answered. “If a light goes out, my favorite sensor is the sensor on the light. (It lets us know, within fifteen minutes, that the light went out and what type of light it is, so we can get a replacement and fix it before anyone notices.)”
Navigating the challenges
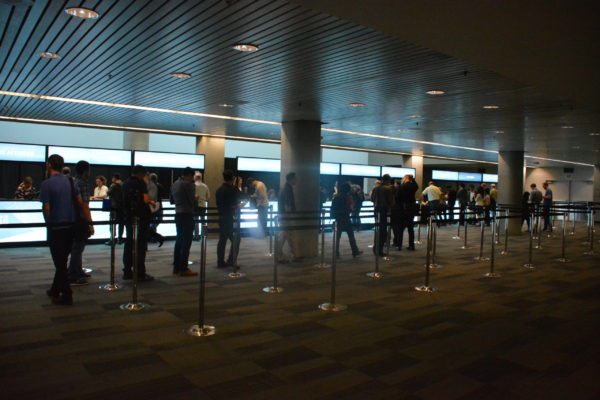
Attendees check in at registration desk for Sensors Expo, 2018.
Jesse said about 130 customers had, so far, signed up for the new connected LED lights.
Asked about privacy concerns with the sensors, he told Matt, “We’re also seeing a reduction in crime (where the smart lights are deployed). What we’re seeing is, people will compromise a little bit of privacy (for better public safety).”
However, Jesse said, the obstacles to the project include economic considerations–and education.
“Just understanding what (the choices are for the smaller towns),” he explained. “How to gather the data and make it available to elected officials? It’s overwhelming.”
Understanding a town’s specific needs, he said, was crucial.
“The last thing you want to do is (implement technology) that turns into birds’ nests or collects cobwebs.”
Jesse said NYPA, as a public authority, is only allowed to work in New York State. But they have a far-reaching vision–and he invited any other state municipalities to contact him if they’d like more information.
“We want to see all street lights (connected) throughout the country.”
Sensors, crops and AI
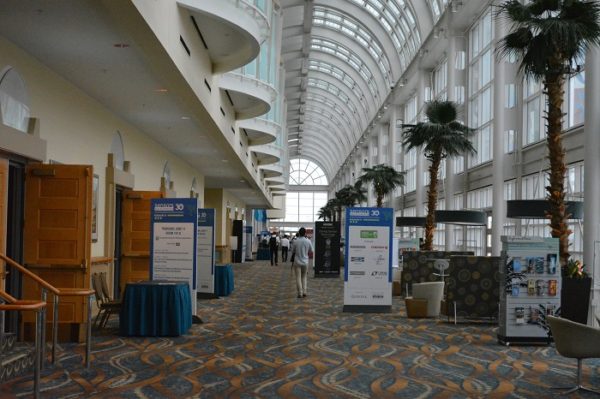
Sensors Espo and Conference, Long Beach Convention Center, 2015.
During Sensors Innovation Week, each day’s program was comprised of two ninety-minute sessions. Those, in turn, contained multiple fireside chats, keynotes and panels on current developments in sensing technologies.
In other words, one post can’t possibly do justice to all the speakers, and the information they shared.
However, one personal favorite event took place during Friday’s final series, entitled “AI and Machine Learning: Making Sense Out of Sensor Data”.
Once again, Fierce Electronics Group Content Director Karen Field moderated a discussion panel. Panelists offered their perspectives on the use of artificial intelligence in the agricultural area.
“What was a memorable ‘aha’ moment for you?” Karen asked the panel.
“Our partner is Mother Nature, and she’s very volatile,” answered John Deere Strategy Lead for Digital Solutions Sona Raziabeegum.
“The bigger ‘aha’ for me: that particular AI solution has to handle itself very well. It’s important to zoom out your perspective. How is that AI going to work in the customer’s workflow (and integrate with existing systems)?”
“I’ve been working in the semiconductor industry for more than twenty years now,” said ST Microelectronics IOT Sensors, RF and Cloud Application Director Francesco Doddo.
“My aha moment…sensors and microcontrollers were really super efficient. So it’s easy to bring that into the cloud from wherever you are.”
“I started working with AI back in the 1980s,” said The Aerospace Corporation Senior Project Leader – Embedded Systems Architect Mike Anderson. “{Back then), AI had promised so much, and failed to deliver much of it.”
Mike said his particular “aha” moment came while working with a government agency. Their AI application: determining which terrorist group was responsible for a particular act of terror.
(“That was the first time I saw artificial intelligence work effectively”), Mike said.
Sensors on the farm
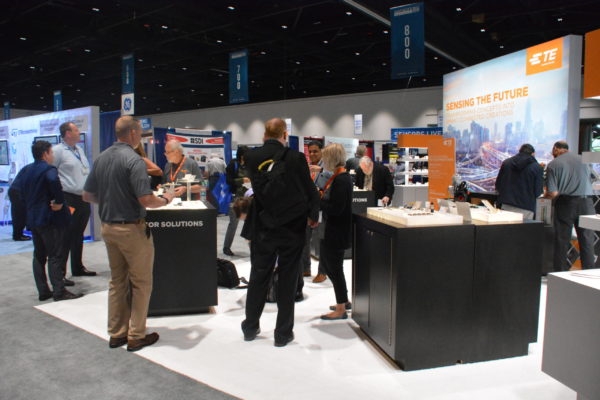
On the Sensors Expo floor, San Jose, 2019.
“In agriculture, (AI) is actually a reality,” Sona said. “We have commercial products available.”
She outlined two use cases.
The first, a “combine advisor” launched a few years ago, works as a farmer drives a combine to harvest grain. With traditional farming, the operator cannot see the quality of the grain being harvested while operating the machinery.
However, Sona explained, with the combine advisor, RGB cameras in the combine’s grain tank photograph the crops being harvested. The cameras take images at a rate of two prints per second. Then, neural maps inside the cameras determine the quality of the crops, in real time. The AI will automatically adjust the machine controls, if the grain is damaged or the combine is picking up debris.
“…You’re essentially having the combine run in cruise control, and alleviating a lot of stress on the operator,” Sona commented.
Sona said this AI-enhanced combine currently has close to 70 percent adoption in the market.
The second use case, she said, is the “See and Spray”, a product not currently on the market. This is a self-propelled sprayer, used to spray both herbicides and nutrients to crops.
“..We have cameras on a 120-foot boom,” Sona explained. “The cameras are taking (one picture every fifteen milliseconds. That’s half the time it takes you and me to blink. And there’s a neural map on the edge, that determines, on the spot, whether what it’s looking at, is a weed or an actual crop.)
“…Depending on the determination that it’s a weed, it can squirt herbicide, the size of a postage stamp, just at the weed. And so that results in up to a ninety per cent reduction in herbicide use.
…”The truth of the matter is, our farmers are using neural maps today, and that’s pretty exciting…AI in agriculture is not the future. AI is the ‘now’ of agriculture.”
Considerations and challenges
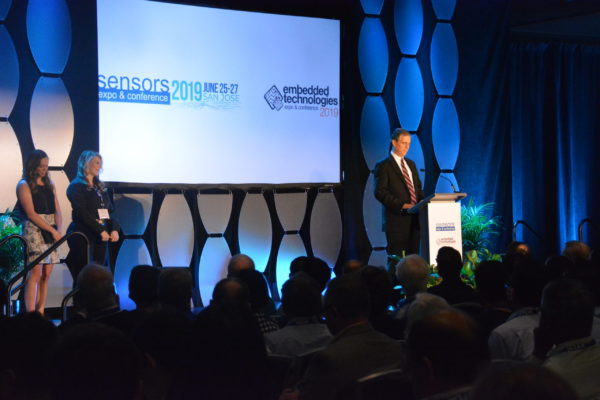
Awards for innovation, at Sensors Expo and Conference, San Jose, 2019.
The panel explored some of the variables that could affect introduction of machine learning into agriculture.
Sona referenced a customer’s’ learning curve as a determinant to where AI should be applied.
“If they have to…learn a lot of things, if the technology’s too complicated or just doesn’t fit with (their) workflow, (it’s) not going to get adopted,” she said.
For that reason, Sona added, the “See and Spray” was currently in field trials, to determine what works, and doesn’t work, for John Deere’s prospective customers.
Battery life was a concern which Francesco mentioned.
“In the past years, the wearable space has been very dominant,” he said.
“…Wearable and hearable devices, those are all battery-operated. …So what you want to make sure, is that machine learning…is done at very low power consumption.”
“Not quite a success”
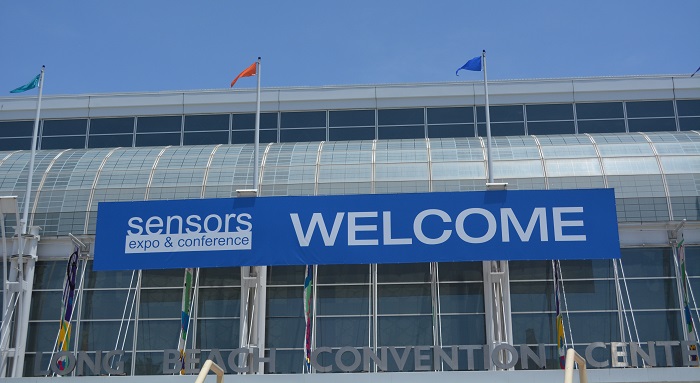
Banner welcomes attendees to Sensors Expo 2015, Long Beach.
The discussion moved on–to lessons learned.
“I think people come to these events hoping to tap into your experiences, and hopefully learn something that they can avoid, themselves, in the future,” Karen said.
” (Is there)… a situation that you could describe where you guys just blew it? Something in the process that you need to go back and reevaluate?” Karen asked.
“I actually would like to tilt the perspective on this whole “failures and mistakes”…I look at every failure or every challenge as something…” Sona begam.
“Not quite a success,” Mike interjected. “It’s not a failure, it’s just not quite a success.”
“Exactly, Mike,..if you just are focused on success you probably are not trying hard enough,” Sona answered. “…Real-world data is really messy….there’s a lot of great learnings along the way.”
Sona shared one “not quite a success” experience with data collection for AI for John Deere’s self-propelled sprayer.
“We had to create an initial critical mass of images, (of weeds and plants) to actually have a training model,” she said. “…These images didn’t exist in a shell somewhere that we could just go buy. We actually had to go out and data collect ourselves..
“Initially, we thought we could just put cameras on our machines, but there’s a limit to how may times you can drive these tens of machines through the fields without impacting land quality.”
In addition, Sona said, seasonality was a factor. So was collecting data points from different parts of the country. Putting cameras on rigs, and transporting those rigs on flights to other areas, proved unworkable.
“We finally ended up with these backpacks. that people could just have on their back, and walk through the field, connecting initial data,” Sona said. “So that was an interesting learning journey.”
Lessons from the field
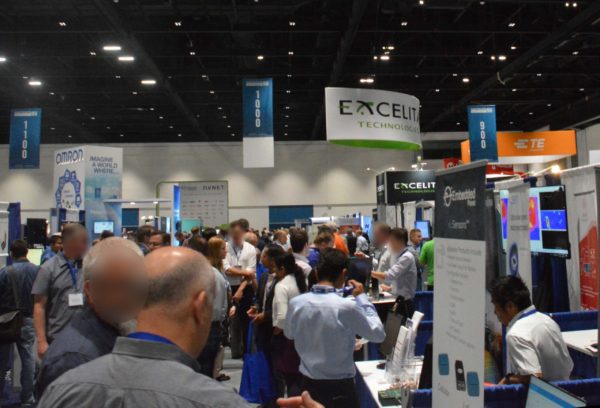
On the expo floor at Sensors Expo and Conference, 2019, San Jose.
“…(AI) is still a new field for a lot of customers,” Francesco said. “I believe there are two main things that are pretty important.
“First of all, make sure that your team is equipped with the right skill set. Which are…data scientists, but also electrical engineers…and they can work together properly. Because the data scientist probably has no idea how the hardware works, the electrical engineer has no idea how a problem can be stated from a data-science perspective.
“…Usually, this is what we see when we work with companies. Most of them, they’re either coming from one side: no knowledge of the hardware, or the other side: no knowledge of the data science. And unfortunately those needs are super important.
“And then the other point,… (is) having a clear problem statement. Which means a lot of things.
…”How do I make sure that that goal is realistic?…Once I get that goal, (how do I monitor it?)…How do I define my data collection? (When can I say my data collection is good enough?) How do I clean up my data…and what do I expect, in terms of performance from those data?”
“Believe it or not, this is usually something that…you learn along the way.
“…It’s going to be like this for maybe forever, but what we can learn, is that if we have a clearer way to define our problem, and also to measure that problem, the time to market is dramatically reduced.”
Expectations versus reality
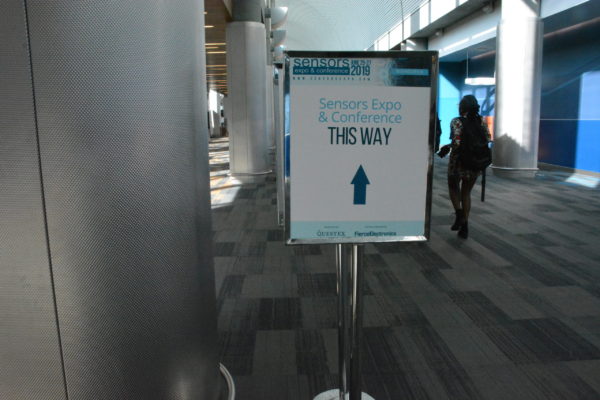
Attendees head for Sensors Conference 2019, San Jose.
“One of the things we really haven’t talked about at this point, is management of expectations,” Mike said. “As engineers, we want to be able to promise the world, and the reality is, you can’t deliver the world.
“Especially when it comes to machine learning. There are a lot of misconceptions about what it can and cannot do.
“We hear people say, ‘Oh, well, it’s got the intelligence of a two-year-old now. It should certainly be able to be a ten-year-old within another year.’
“Well, yes. It has the intelligence of a two-year-old, in the sense that a two-year-old can tell the difference between a can of Coke and a kitten. That doesn’t necessarily mean that’s a useful skill, but it’s certainly something that most neural networks can do.
“You have supervised, …unsupervised, …feedback learning loops, (and all kinds of different characteristics in different algorithms). As (engineers) we have to be able to manage expectations from our management. Because they’ have seen too many sci-fi movies, …and they think that it’s suddenly going to be able to solve all their problems if they just introduce AI.”
Moving forward
Wrapping up the panel–and Sensors Innovation Week– Karen asked a final question:
“How do you keep your customers from trusting their AI more than they really should?”
“Management of expectations,” Mike repeated, laughing.
“You can have (artificial intelligence) where it’s very good at being able to predict the color of the traffic light, as you’re coming up to it. But that’s a fairly simple problem. Are you going to be able to predict the cross traffic, and whether or not you’re going to have somebody run through a red light?
“You have to realize, the AI, the ML, has limitations (and data scientists and engineers need to be honest about those limitations up front).”
“It is a trust,” Sona added. “(We’ve been looking at demystifying the ways the technology is actually operating, and keeping the human in the loop.)”
“Keeping (the customer) in the loop, …and essentially bringing them along for the journey. I think that’s very critical.
…”And then, essentially giving them control to intercede along the way.
“…It’s a really great question and (it shouldn’t be a question of ) either trusting too early, or not trusting at all.”
Like to know more about artificial intelligence? Another free virtual event, “Fierce AI Week”, is coming up August 10th through August 12th! Here’s the link for information.